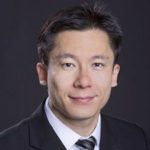
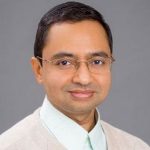
Xiao Qin, professor, civil & environmental engineering and director of UWM’s Institute for Physical Infrastructure and Transportation, was awarded $99,951 in April to develop a web-based application (called Crash Information Extraction, Analysis and Classification Tool, or CIEACT) to improve data analysis related to highway crashes. Qin will collaborate with Rohit Kate, associate professor, computer science.
The award comes through a traffic safety information system improvement grant from the National Highway Traffic Safety Administration (NHTSA) of the U.S. Department of Transportation, through the Wisconsin Department of Transportation. NHTSA oversees highway safety issues, including human behavior that contributes to crashes (i.e. reckless driving, DUI, improper cellphone use) and data related to them.
The project aims to improve Wisconsin’s highway safety analysis by using technology to capture and analyze valuable information from unstructured data, such as police officers’ narratives, that relate to human behavior. Researchers will use machine learning and text mining techniques for automatic text analysis and feature extraction.
The problem with “unstructured” data
Eighty percent of worldwide data is unstructured, meaning it’s in text documentation, photos, audio and videos, Qin says. “Unstructured data can’t be easily stored in a database,” he says. “And even if it is stored, it has attributes that make it a difficult to edit , query and analyze, especially on the fly.”
Human behaviors contribute to between 80 and 90 percent of crashes and can fall into this category, meaning they are not adequately captured in current analyses used to guide highway planning and design and to make driving safer, Qin says.
Law enforcement officers’ narratives can use different words or phrases, which presents a challenge for traffic safety engineers when querying specific terms. And while engineers often manually review the reports to search for causes and contributing factors for remedial actions, the process is labor intensive, and the review quality is inconsistent, as it is subject to the reviewers’ experience and judgement.
Applying intelligent algorithms to police narratives
To extract data from police narratives, researchers will implement intelligent algorithms (i.e. natural language processing, text mining, statistical modeling) that they have already designed and tested.
Qin hopes that the functions and analysis offered by CIEACT will provide safety practitioners and professionals with maximum and quick access to information stored in the texts of crash narrative, substantially reduce crash report review time, and significantly improve review quality and consistency.